Previous Article
Global Sportswear Brand — Product Engagement Analytics
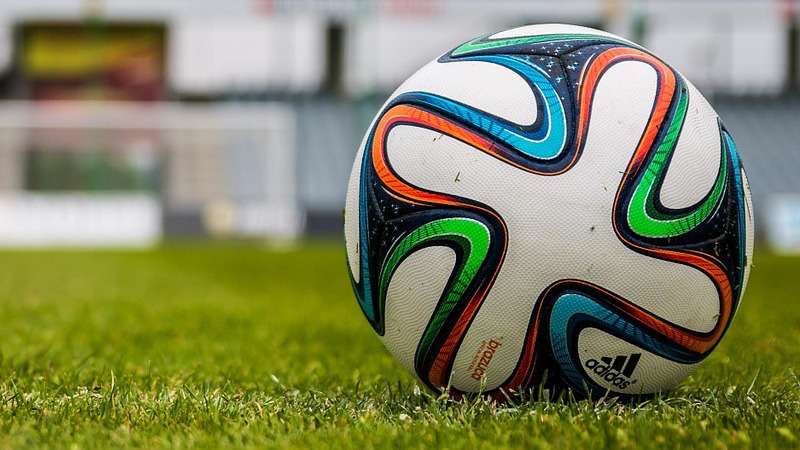
Summary
A major global sportswear brand needed to understand why users were churning from their app at such a high rate and how to create more relevant app features.
Silicon Valley Data Science developed methods to understand the patterns of customer behavior so that the client could make better marketing and product development decisions.
Background and Business Problem
Product analytics allow businesses to identify patterns in users’ interactions with their app to inform its future development and marketing. This is key to making decisions based on how users are actually using a product rather than how they or the business think they are using it, leading to increased user satisfaction and engagement. A major global sportswear brand needed help building and proving its product analytics capability to support the growth of their new digital products (i.e. mobile apps).
Our client is growing their mobile presence by developing a suite of apps complementing its products (e.g. running, training, soccer). However, many of their apps faced a very high user churn rate. In this particular example, their running app faced an undesirably high churn rate within the first 10 runs and there was very little visibility into why users were churning so quickly. The company wanted to use data-driven insights based on how users are using the app to validate assumptions and drive the product roadmap.
As there was an opportunity to use product analytics to arm the product development team with user insight, the company engaged SVDS to investigate a number of hypotheses around the types of incoming app users—who the app was under serving (and why they were churning), and who the app was serving well (and how they could continue to increase value).
Solution
A base assumption that our team operated on during the investigation was that a user will continue to use the app if they find value in their use of the app, and if they continue to run.
Using this base assumption, we investigated ways in which we could derive user interest and intent through the workflow of their first visit. Using interactions recorded during their first visit, we conducted unsupervised learning to identify the intent of the user upon download of the app.
We then assessed how these first visit and run behaviors related to retention. A single recorded run can signal if a user is already an active/experienced runner or if they are a beginner. Beginner runners are more likely to stop using the app because they are unable to establish a habit of running, while churn of already-regular runners indicates the user is not finding value in the app. Analysis of users’ first visit segments, first run segments, and time until churn provided insight into what type of runners are beginning to use the app, why they are interested in using it, and whether they perceive value in the app for their intended use. We then presented to our client a set of insights based on initial exploratory data analysis and the analysis of the types of users that fall into each first visit and first run segment and combination thereof.
One of the first insights revealed that the majority of the users using the app are actually not the intended target audience. As the workflow and programs offered on the app were a mismatch with the actual users of the app, the users churned very quickly as they did not find value in using the app. This demonstrates the importance of tying product analytics closely to product development so as to make informed decisions on features of the app.
At the end of the analysis, we presented a final set of insights that communicated patterns observed around density, retention, feature use distinction, and run type consistencies of the users. By better understanding the types of incoming app users, the company can use data-driven insights to bring value to them and create stronger engagement with their customer base.